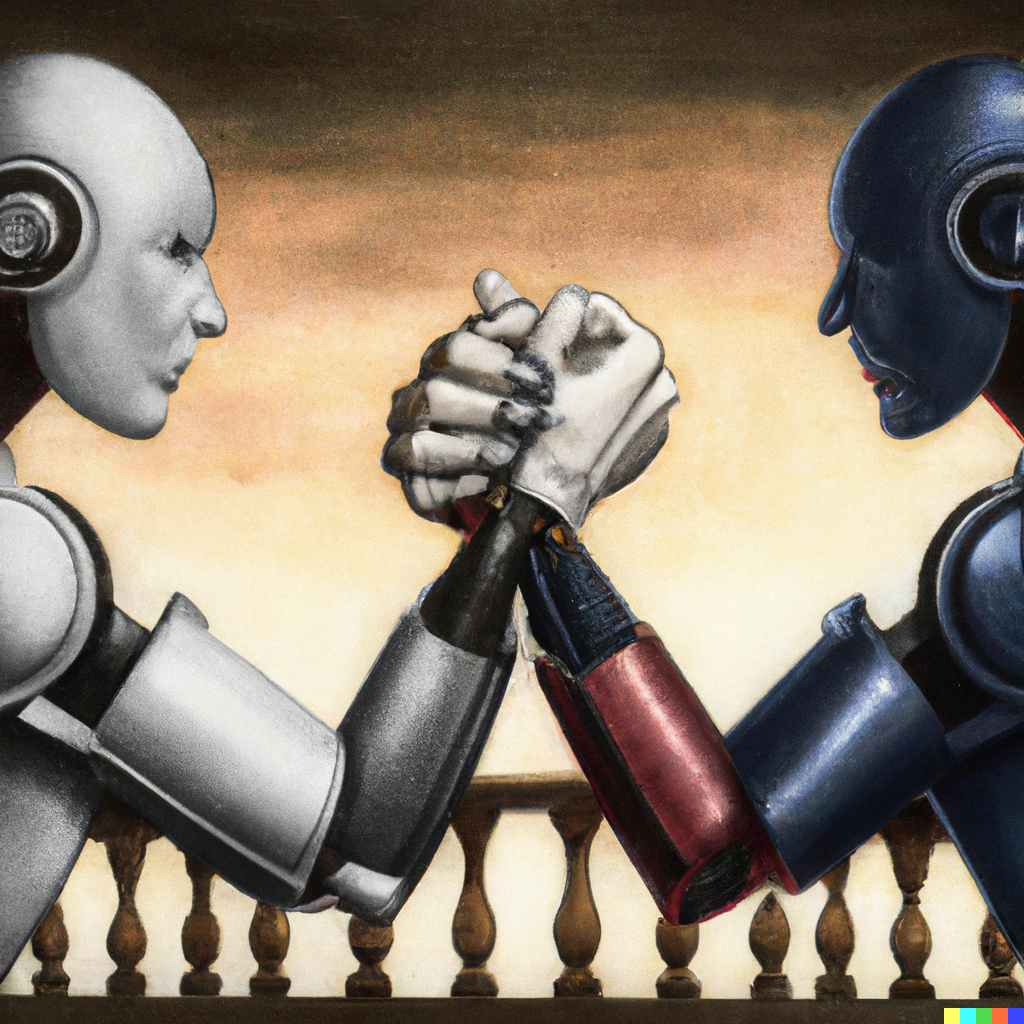
Sponsored By: Masterworks
Masterworks is the award-winning platform helping 550,000+ invest in shares of multi-million dollar art by iconic names like Picasso, Basquiat, and Banksy.
Over the last few months, I’ve been obsessed with AI—chatting with AI researchers in London, talking product development with startups in Argentina, debating philosophy with AI safety groups in San Francisco. Among these diverse individuals, there is universal agreement that we are in a period of heightened excitement but almost total disagreement on how that excitement should be interpreted. Yes, this time is different for AI versus previous cycles—but what form that difference takes is hotly debated.
My own research on that topic has led me to propose two distinct strategy frameworks:
- Just as the internet pushed distribution costs to zero, AI will push creation costs toward zero.
- The economic value from AI will not be distributed linearly along the value chain but will instead be subject to rapid consolidation and power law outcomes among infrastructure players and end-point applications.
I still hold these frameworks to be true—now I’d like to apply them to six “micro-theories'' on how AI will play out.
This research matters more than typical startup theory. AI, if it fully realizes its promise, will remake human society. Existing social power dynamics will be altered. What we consider work, what we consider play—even the nature of the soul and what it means to be human—will need to be rethought. There is no technology quite like this. When I discuss the seedling AI startups of today, we are really debating the future of humanity. It is worthy of serious study.
1. Fine-tuned models win battles, foundational models win wars
My consolidation theory posited that the value chain for the AI market looked something like this:
In contrast with traditional markets, each building block of the AI chain is intermingled with the other components. Rather than the normal linear product development (a steady refinement of raw materials until a product is created) AI is a snake eating its own tail because the various components feed off of each other. For example, trying to improve the data you receive will also simultaneously improve access points—it is a self-referencing series of feedback loops. This is particularly true in the case of foundational models (models that attempt to do one broad task well, like text generation) versus fine-tuned models (where they tune foundational models to a specific use case, like healthcare text generation).I’ve since heard tales of multiple startups that had spent the last few years fine-tuning their own model for their own use case. Thousands of hours devoted to training, to labeling specific data sets, to curation. Then, when OpenAI released GPT-3, it was an order of magnitude better than their own version.
Are you concerned about historic inflation? Your stock portfolio in meltdown? A collapsing real estate market? Then you should know about this $1.7 trillion alternative asset – its prices outpaced the stock market by more than 2x over the last 26 years.
Fine art. That’s right, art. It's easy to get started with Masterworks, the award-winning platform helping 550,000+ invest in works by world-renowned artists (think Picasso, Basquiat, and Banksy) without breaking the bank.
While others were panic selling at double-digit losses, Masterworks’ recent exit delivered a 33.1% net return*.
Every readers can skip their waitlist to join.
Fine-tuning makes requests much cheaper for narrow use cases. In the long term, it's not necessarily about fine-tuning beating foundational model performance but more like being deployed for narrow use cases to lower the cost of prompt completion. However, this can only happen when companies decide to train their own models. At a certain level of scale, it’s faster and easier to rely on someone else.
You can think of the AI model race as something like the graphic I made below. Fine-tune models can gradually improve over time, while foundational models appear to take step-changes up.
This graphic shows both strategies converging at the same point, but depending on your beliefs, you could have one outperforming another.
If this is the race, the natural question is: who will be the winner?
2. Long-term model differentiation comes from data-generating use cases
The current AI research paradigm is defined by scale. Victory is a question of more—more computing power, more data sets, more users. The large research labs will have all of that and over time will do better.
However, there is one instance where the graph will look like this:
I drew the fine-tuned performance as a series of little circles, which represent data loops. Data loops occur when an AI provider is able to build feedback mechanisms into the product itself and then use that feedback to retrain the model. Sometimes they’ll do so by utilizing their own foundational model, sometimes they’ll fine-tune someone else’s model for their own use case. I think that this will require a model provider to also own the endpoint solution, but I’m open to being wrong on this point. Think of it as power-ranked 3) small, specialized model 2) large general model, and 3) large specialized model. The issue with stack rank 3 is that these can only exist if you make them yourselves. Startups that can capture that model to output to retrain model loop will be able to build a specialized winner.
3. Open source makes AI startups into consulting shops, not SaaS companies
For many years, most people assumed that AI would be a highly centralized technology. Foundational models would require so much data and computing power that only a select few could afford it. But that didn’t happen. Transformers, the math driving a lot of this innovation, didn’t require labeled data as previously thought. Look at what’s happened with image generation.
Source: State of AI Deck, 2022.
An even greater Cambrian explosion has happened in text generation.
This is an industry rooted in academia. Whenever a company launches a new product, they typically publish an accompanying article in a major scientific journal outlining the math, data set, and process they used to create the model. This is good for science, bad for business. Since so many of these models train on similar data sets and the math is all widely available, the only thing holding these companies back is compute power. Essentially anyone can build a copycat if they have sufficient skills and resources.This dynamic can result in rapidly eroding market power. For example, DALL-E 2 started with a limited beta that was restricted in its use. When Stable Diffusion, an open-source DALL-E clone, launched, Open AI had to remove the waitlist and usage restrictions within a month.
I wondered how these open-source AI companies would function, so I chatted with Jim O’Shaughnessy, executive chair of Stability.AI, the company behind Stable Diffusion.
“The actual value in AI shakes out in kind of the white glove service for the biggest content providers, like Disney and Sony,” O’Shaughnessy told me. “Even all the game makers in the world have woken up to the fact that they need to have AI for their content. That's going to require a white glove service, because you're going to have to continually tune the model as it learns on the new content. However, that tuning has got to be closed. They don't want their IP going out into the world. Bespoke foundational models is the service. I think it's going to be a huge market.”
Offering customized instances of open source software isn’t without precedent in technology markets. Redhat built a multi-billion-dollar business helping companies manage their Linux technologies. However, these will look more like consulting companies than software businesses, and margins and profit will follow accordingly.
Open source also puts downward pricing pressure on model providers that sell access to their model via API. When you’re competing with free, you almost always have to compromise by being cheap. I asked one startup founder, whose AI product is currently being used by thousands of customers, what was most surprising about building off of OpenAI’s API. “It is stupid cheap,” the founder (who requested anonymity so that OpenAI wouldn’t raise their prices) told me. “We are only spending a few hundred bucks a week.”
OpenAI has circumnavigated this pricing pressure by taking equity stakes in the most promising startups with its $100M venture fund. Arguably, they will be best positioned to take AI bets of all venture capital firms: they observe which companies are experiencing a rapid acceleration in use of their products and call them. Hypothetically, this could work, but who knows.
4. Most endpoints compete on GTM, not AI
When we launched our own AI product Lex, I expected to maybe get a few thousand signups. Instead, we hit 60K sign ups within 23 days of launching. We did no marketing besides a tweet. Even more remarkably, my colleague Nathan built it himself over a few weeks of nights and weekend hacking. The AI is done via GPT-3.
Within two months of launching, not only are there multiple competitors that we know of, there is an entire course dedicated to copying what we built! The course even calls this out:
There are currently 492 copycats, cough, course participants building Lex knockoffs. They will be able to access identical AI building blocks as us.
Rapid success followed by the rapid emergence of copycats will hold true for other companies. According to several investment memos I saw, Jasper.AI, a similar AI writing and content tool, went from $0 to ~$40M in ARR within eight months of launching their AI product—a pace would put them on track as one of the fastest-growing SaaS businesses of all time. Soon after their success, a large swath of competitors, all relying on the same open-source or AI models, raised venture funding to pursue similar opportunities.
It will be incredibly difficult for customers to measure product performance to compare these startups. You can get efficacy benchmarks from vendors, but those are tough for the average employee to test or even understand. A lot of the purchasing decision ends up being driven by GTM strategy instead of pure vendor comparison. The question of which “AI” startup wins their endpoint is more a matter of sales and marketing and vibe than model performance.
AI is marketing gas. People are hungry for products that can perform these tasks. However, the winners will be determined by software questions, not AI ones. For endpoints selling AI services, they will either need to fully own fine-tuned models or compete on the typical attributes of a SaaS startup.
For those startups that are competing on the basis of SaaS, the competitive advantage goes toward companies that already have inherent distribution or product capabilities. It would be much easier for a large company to integrate AI into their existing products than for startups to build competitive full-suite products from scratch. Microsoft, Canva, Notion, and others have already replicated some of these startups' capabilities within their existing product suites. I expect every major software provider to integrate generative AI into their products over the next six months.
Note: Credible sources have told me that GPT-3’s successor GPT-4 is far beyond what people are expecting. It’s currently in testing with a variety of OpenAI’s friends and family, and it will leave most fine-tuned models in the dust.
Online reading app Readwise recently launched an AI tool that included capabilities like:
One developer was able to build 10-plus AI features in about three weeks. Cofounder Daniel Doyon told me, “You can launch AI features pretty easily, but for someone to replicate our Reader it will take five engineers about a year of hard work.”
Most AI startups are just SaaS startups wearing a tophat.
Or as the Jasper.AI CEO put it:
5. AI will not disrupt the creator economy, it will only amplify existing power law dynamics
In September 2021, I proposed a theory for why non-professional creators were able to outperform historically powerful media shops. Because there are so many of them, creators in aggregate are more likely to make “edgy” content that performs well under algorithmic amplification paradigms.
There is a reason people like Joe Rogan, Andrew Tate, or take your choice of edgy influencer can consistently outperform places like CNN. They have more freedom to experiment and push boundaries. This content, in turn, outperforms traditional media shops because extremes drive clicks, which drive revenue. And if Rogan or Tate ever stepped away, other edge-lords would take their place because there is an infinite supply of people who want to be YouTube stars.In a world where AI pushes content creation costs to zero, what happens? Conventional wisdom is that we will all be consuming custom-generated AI content meant just for us within the next couple of years. In a world of infinite choice, AI will, supposedly, make stuff that is meant just for you that is better than anything else out there.
I disagree. Some low-brow, non-social content may be replaced. But in a world where content is basically free to make, what matters is distribution. Creators who properly utilize AI tools to make better content faster will be able to build a critical mass of fans. The world of digital media is already one where .01% get all the revenue; AI will exaggerate that dynamic even further.
Furthermore, content is an inherently social experience. There is a reason subreddits form whenever a new artist gets a fanbase. When you experience amazing content, you want to share it.
There is already more content created than we can ever consume. My wife and I have a backlog of movies and TV shows that is hundreds of hours long. We’ll never finish it. Will it matter if AI adds more stuff to that backlog? The ability to break through and require me to pay attention requires a level of fandom and marketing that cheap content can’t match. Expect to consume more from your favorite creators and less from everyone else.
6. Invisible AI will be the most valuable deployment of AI
In my proposal of the AI value chain, I argued, “The most successful AI company of the last 10 years is actually TikTok’s parent company, Bytedance. Their product is short-form entertainment videos, with AI completely in the background, doing the intellectually murky task of selecting the next video to play. Everything in their design, from the simplicity of the interface to the length of the content, is built in service of the AI. It is done to ensure that the product experience is magical. Invisible AI is when a company is powered by AI but never even mentions it. They simply use AI to make something that wasn’t considered possible before, but is entirely delightful.”
AI at mass deployment breaks many of the mental models for how computing works. In pre-AI world, our creative output was scarce and precious. In AI robot world, creative output is infinite and overwhelming. This changes what computing is at a fundamental level—the structure of documents, the purpose of outputs, all of it altered. Think of a digital artist. Is the art the output or the process going into the output? Is the art the prompt someone gives to the AI?
Even something as mundane as file management can be reimagined. The typical file structure is a series of cascading folders.: photos go to July photos, go to the Fourth of July, etc. In a world where AI can generate hundreds of photos every minute, what are you supposed to do? SOOT uses AI to auto-tag images by their components so you can dump them all into a visual space and sort them by their content (as well as time and variety of other attributes). For example, you can look for swimming pictures…
...and the AI will find and sort them into the ones most like your description.Lots of companies do this, but it’s even cooler when you mix those search capabilities with generative AI. Let’s say you were looking to create a new arrow icon and had a reference image. Click on it and, in the modify field, write that you’re looking for an arrow in the style of Virgil Abloh.The AI will create a bunch of variations.
Contrast this with the previous process of file management and image creation, where designers would flip back and forth between Figma, Photoshop, and Dropbox just to manage a few images.
This product is something that is only possible with AI. I don’t know what all of the startups will look like, but when you mix an invisible AI backend with AI generative capabilities, magic can happen. AI products can’t simply be model-deployed to existing use cases. AI products win when they enable entire new modalities of digital interactions.
This may all be pointless
These startups, these games of productivity software, matter, because they lay the bedrock for how we approach building AI for the next 10 years. We’re in the formative period. The frontlines of the future are happening right in front of us.
You’ll note that I didn’t mention the singularity or artificial general intelligence—ideas that are worthy of their own piece. If or when AGI occurs, it will be the most important technology mankind has ever invented. It also may render everything I ever wrote on this topic entirely moot.
Find Out What
Comes Next in Tech.
Start your free trial.
New ideas to help you build the future—in your inbox, every day. Trusted by over 75,000 readers.
SubscribeAlready have an account? Sign in
What's included?
-
Unlimited access to our daily essays by Dan Shipper, Evan Armstrong, and a roster of the best tech writers on the internet
-
Full access to an archive of hundreds of in-depth articles
-
-
Priority access and subscriber-only discounts to courses, events, and more
-
Ad-free experience
-
Access to our Discord community
Thanks to our Sponsor: Masterworks
Get unparalleled access to invest in the same contemporary art that you see in the most famous private collections, galleries, and museums around the world – for just a fraction of the price of the entire work.
See important Reg A disclosures.
Comments
Don't have an account? Sign up!