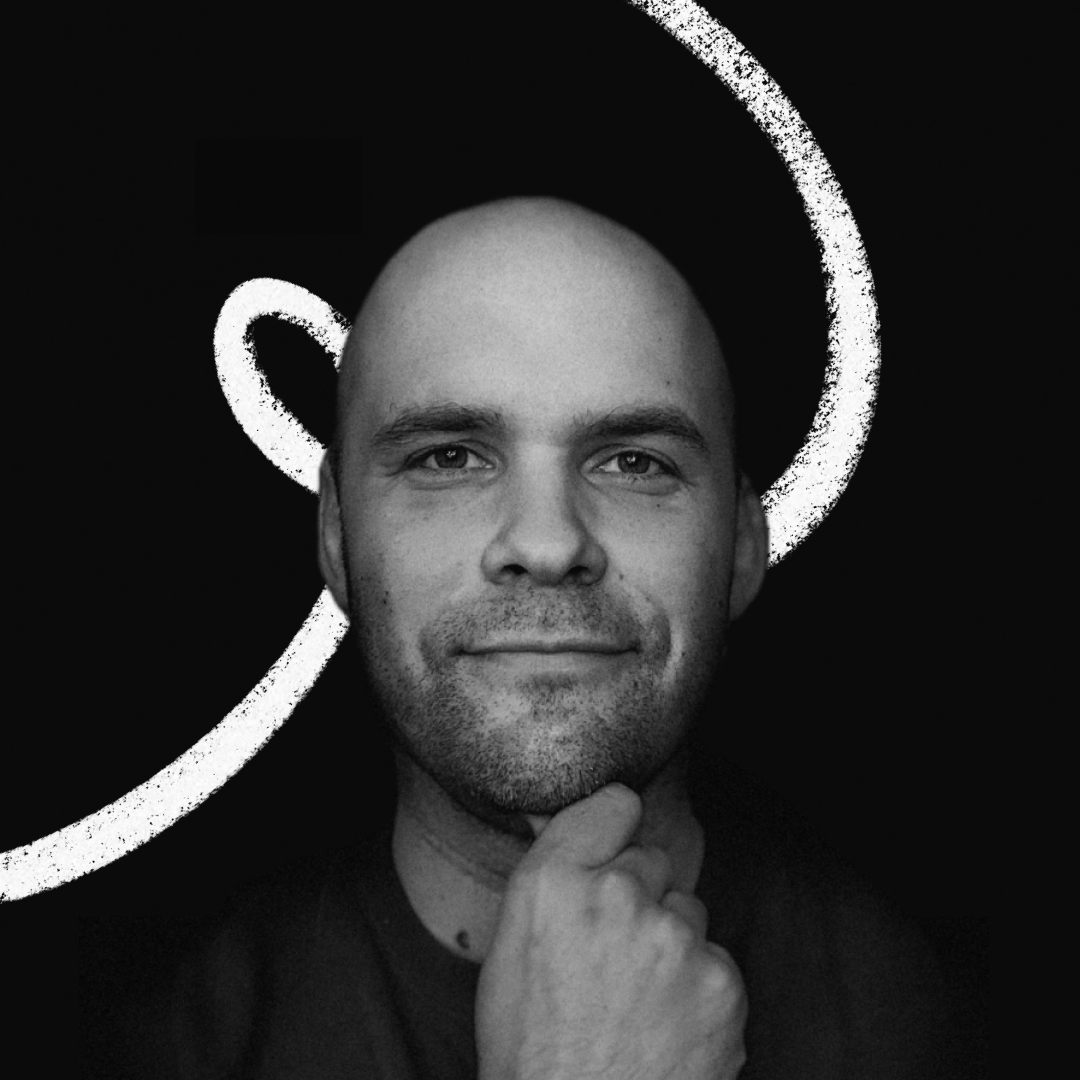
What Do LLMs Tell Us About the Nature of LanguageâAnd Ourselves?
An interview with best-selling sci-fi novelist Robin Sloan
June 12, 2024
TL;DR: Today weâre releasing a new episode of our podcast AI & I. I go in depth with Robin Sloan, the New York Times best-selling novelist. We dive into what LLMs and their internal mechanics tell us about the nature of language and ourselves. Watch on X or YouTube, or listen on Spotify or Apple Podcasts.Â
One of my favorite fiction writers, New York Times best-selling author Robin Sloan, just wrote the first novel Iâve seen thatâs inspired by LLMs.
The book is called Moonbound, and Robin originally wanted to write it with language models. He tried doing this in 2016 with a rudimentary model he built himself, and more recently with commercially available LLMs. Both times Robin found himself unsatisfied with the creative output generated by the models. AI couldnât quite generate the fiction he was looking forâthe kind that pushes the boundaries of literature.
He did, however, find himself fascinated by the inner workings of LLMs
Robin was particularly interested in how LLMs map language into mathâthe notion that each letter is represented by a unique series of numbers, allowing the model to understand human language in a computational way. He thinks LLMs are language personified, given its first heady dose of autonomy.Â
Robinâs body of work reflects his deep understanding of technology, language, and storytelling. Heâs the author of the novels Mr. Penumbraâs 24-hour Bookstore and Sourdough, and has also written for publications like the New York Times, the Atlantic, and MIT Technology Review. Before going full-time on fiction writing, he worked at Twitter and in traditional media institutions.Â
In Moonbound, Robin puts LLMs into perspective as part of a broader human story. I sat down with Robin to unpack his fascination with LLMs, their nearly sentient nature, and what they reveal about language and our own selves. It was a wide-ranging discussion about technology, philosophy, ethics, and biologyâand I came away more excited than ever about the possibilities that the future holds.
This is a must-watch for science-fiction enthusiasts, and anyone interested in the deep philosophical questions raised by LLMs and the way they function. Hereâs a taste:
- Cultivate a note-taking habit. Robinâs note-taking habit inspired some of his novels, and he believes that building a repository of things you find interesting unlocks new avenues of creative pursuit. âThe great thing about keeping notes and trying to cultivate a sense for that stuff that just appeals to you in a hard-to-describe way is [that] youâre essentially writing the perfect blog for yourself,â he says.
- Prompt LLMs to mimic your voice. If you can articulate the tone and style of content that you want to create, Robin thinks LLMs would excel at generating output that meets your expectations. The âtrick of fitting [LLMs] into a styleâ by prompting the model to write in a specific genre or format like a âmurder mysteryâ or a âbusiness memoâ is âreally impressive.â
- The ingredients of great fiction writing. Through his experiments with AI, Robin discovered that language models couldn't generate exceptional creative writing because they were trained on a âfuzzy cloudâ of generic data. He believes that truly great writing lies âway out at the edge of that probability cloudâŠ[itâs] the stuff that expands the frontier of what we thought could be written.â
- Balancing known inputs and fresh outputs. Robin is unsettled by the fact that the sheer volume of data used to train commercial LLMs makes it impossible to know exactly what information they contain. He wishes âthere was a system where I was able to sayâŠI know what's in [the LLM]...and now it's going to operate in this living, organic, unpredictable way,â a concept materializing with ethically trained models.
- From code to cognition. Robin finds the idea that LLMs may have improved reasoning abilities due to being trained on vast amounts of code intriguing because it raises questions about the linguistic properties of programming languages. â[Code] is a bridgeâitâs a way for us to think in a more machine way, and we express that in these linguistic terms,â he muses.
The philosophical underpinnings of how LLMs workÂ
The narrator in Moonbound frames the central question of the human race as, âWhat happens next?â These are Robinâs thoughts on the query and its philosophical implications in the context of LLMs:
- Anticipate the future to thrive in the present. Robin says that he uses the narrator of his book to ask, âWhat happens next?â because itâs a fundamental query thatâs âcarved into [his] heartâ. He elaborates that the question is essential for all forms of life, from a âlittle microbeâ to a functioning human being, because it âallows [them] to do things like plan and react to possible dangers and all the other things you can imagine life doing.â
- The philosophical significance of LLMs. Robin believes itâs crucial to consider whether LLMs can be regarded as living beings, given that they also grapple with the central question of what will come next. âIf there are not presently, at minimum, dozens of philosophers, cognitive scientists, and ethicists thinking about this stuffâŠthen the academy is derelict in its duty because these are really rich, interesting questions,â he says.
- LLMs are infusing language with life. While Robin doesnât think LLMs are âbeings,â he is drawn to the idea that these models are the personification of language given its âfirst dose of autonomy.â â[I]t's like you rip language out of our heads and our society sets it up and turns a crank on the side and it starts walking around slowly and weirdly, like one of those little wind-up toys,â he explains.
Robin reflects on the release of Moundbound
As we draw to the end of our conversation, Robin shares his feelings about the launch of his new book:
- Power of the written word. Robin is excited about the bookâs publication so he can witness the way readers interpret and engage with it.âI think [books] literally get into people's heads because they have toâyou didn't just watch Moonbound on a screen, you enacted and rehydrated the eventsâŠin your own language model inside your own head,â he says.
- The interplay between LLMs, books, and dreams. Robin concludes with a pet theory he has about a fascinating link that exists between books, LLMs, and dreams. âI feel that the mechanism of dreaming is very similar to the mechanism of language models, kind of saying, âOkay, well, that's weird, but I'm going to keep it goingâ...and the reason I bring it up is that I think that's also very similar to the mechanism of a novelâmy pitch for novels fundamentally is that they are packaged dreams,â he explains.
You can check out the episode on X, Spotify, Apple Podcasts, or YouTube. Links and timestamps are below:
- Watch on X
- Watch on YouTube
- Listen on Spotify (make sure to follow to help us rank!)
- Listen on Apple Podcasts
Timestamps:
- Introduction: 00:00:53
- A primer on Robinâs new book, Moonbound: 00:02:47
- Robinâs experiments with AI, dating back to 2016: 00:04:05
- What Robin finds fascinating about LLMs and their mechanics: 00:08:39
- Can LLMs write truly great fiction?: 00:14:09
- The stories built into modern LLMs: 00:27:19
- What Robin believes to be the central question of the human race: 00:30:50
- Are LLMs âbeingsâ of some kind?: 00:36:38
- What Robin finds interesting about the concept of âIâ: 00:42:26
- Robinâs pet theory about the interplay between LLMs, dreams, and books: 00:49:40
What do you use ChatGPT for? Have you found any interesting or surprising use cases? We want to hear from youâand we might even interview you. Reply here to talk to me!
Miss an episode? Catch up on my recent conversations with LinkedIn cofounder Reid Hoffman, a16z Podcast host Steph Smith, economist Tyler Cowen, writer and entrepreneur David Perell, founder and newsletter operator Ben Tossell, and others, and learn how they use AI to think, create, and relate.
If youâre enjoying my work, here are a few things I recommend:
- Subscribe to Every
- Follow me on X
- Subscribe to Everyâs YouTube channel
- Check out our new course, Maximize Your Mind With ChatGPT
The transcript of this episode is for paying subscribers.
Thanks to Rhea Purohit for editorial support.
Dan Shipper is the cofounder and CEO of Every, where he writes the Chain of Thought column and hosts the podcast AI & I. You can follow him on X at @danshipper and on LinkedIn, and Every on X at @every and on LinkedIn.
Find Out What
Comes Next in Tech.
Start your free trial.
New ideas to help you build the futureâin your inbox, every day. Trusted by over 75,000 readers.
SubscribeAlready have an account? Sign in
What's included?
-
Unlimited access to our daily essays by Dan Shipper, Evan Armstrong, and a roster of the best tech writers on the internet
-
Full access to an archive of hundreds of in-depth articles
-
-
Priority access and subscriber-only discounts to courses, events, and more
-
Ad-free experience
-
Access to our Discord community
Comments
Don't have an account? Sign up!